Carl Ryden has been a regular attendee at Business of Software Conferences for a long time. His company PrecisionLender has been seeing incredible growth over the past few years, providing a huge amount of value in the banking sector. In this talk from BoS EU 2018, Carl talks about how PrecisionLender use AI to offer a valuable service to their customers, and what he sees as the future of AI.
Slides
Want more of these insightful talks?
At BoS we run events and publish highly-valued content for anyone building, running, or scaling a SaaS or software business.
Sign up for a weekly dose of latest actionable and useful content.
Unsubscribe any time. We will never sell your email address. It is yours.
Transcript
Carl Ryden: I let the music play a little bit longer as my son Peter who is eleven is right here. So he actually picked out the walk on music, big Iron Man fan… What an honor it is to speak in this community. It’s one I’ve been a part of for quite some time. We started our company in May of 2009 with quite literally a blank screen and a blinking cursor. And as we’re working through, building our product, we released it January 1st 2010 and since then we’ve added probably 250 banks around the world. For a while it was about a bank a week and we were in the throes of it in the fall of 2010 when we went to our first Business of Software Conference and the argument I made to my co-founders (I’ve been following Business of Software for a long time even back to when it wasn’t a conference it was just a message board that Joel Spolsky ran back in the day for folks building software companies), the argument I made to them was if it’s not worth a few thousand dollars for us to come together and learn our craft and be better at our craft then we probably ought to get out of business you know. And so we came to each of the BoS Conferences for about nine years and each time we’d come to the conference and we would be faced with some kind of huge problem that we were struggling with. And it seemed almost eerie that each time we showed up there was a talk or a speaker or someone who was speaking directly to the problem we were facing. I often kidded Mark have you bugged our offices or my phone lines or something like that? He said no. Telling our story – the company’s grown really well, we doubled every year, we’re about 120 employees spread around the world now, we’re opening an office over here in the EU, we’ve opened one in Sydney Australia, we have one in Charlotte, North Carolina (if you tell my accent I’m not from around here). But I put on the screen instead of the obligatory chart that kind of goes up and to the right, I put on there our values. This came out of one of the early conferences we came to, BoS conferences in the US. We were struggling. We had made a bad hire. We decided on the trip up there to let this person go and so we set about how do we not ever let this happen again. And we saw talk by a guy named Mikey Trafton (who’s coming back to BoS USA this year and I’ve become friends with over the years) called “How to build a world class culture in three not so easy steps”: Figure out what’s important to you and pay attention to it were the two not easy steps and the third one… I forget what it was now… But one of his tasks was when you write down your values of your company they should be the only things you’ll ever fire someone for. So when someone asks you Alison how do you how do you know when the red line is crossed, I wanted to scream out “This is how we do it!”. These are the only things you’ll ever fire some or forcing you to be expansive but also very narrow and it also puts you in a case we don’t end up firing for them very much because you hire against them. We punch people in the face with them when they come in the door. The first thing we show employees, it’s the first thing we show prospective customers, it’s the first thing we show prospective investors because if for whatever reason they’re not going to onboard with this they’re not a good fit for us. And the last person folks interview with at our company is me and I spend a lot of time with them. I spend some time with them I say. My job isn’t to assess your capabilities. People smarter than me have already done that or you wouldn’t be in my office. What I’m here to do is tell you that this is who we are and you’re either going to fall in two camps. You’re gonna go I love that. I’ve been waiting for this my entire life or you’re going to go I’m scared to death by that, I’m gonna be exposed. Either one is a perfectly wonderful outcome for both of us. So I just let him know we mean it and that really helps a lot with the hiring process. So each year I come to BoS and I walk up to Peldi as a matter of ritual and say “You’re my hero”. You know who has two thumbs and is my hero – this guy right here.
So what I will do today is I will talk all about Iron Main Suits and Terminators. We built a product that serves commercial bankers, the commercial relationship managers and they’re an interesting lot. And again we’ve grown quite rapidly. And about two years ago we came up with the idea of building into our system, I call it an A.I.. The word A.I. is I think horribly overused. It means everything and nothing. And it’s really a marketing term which means whatever computers can’t do until they can. So we built Andi who is our intelligent virtual assistant. What I’m would do is take you through three stories. One is a story about a guy whom Doug Engelbart – anybody who Doug Engelbart is? Peldi knows, a few people know – yeah, mother of demos guy. So it’s amazing I discovered him as I was putting together this talk a few weeks ago. And I didn’t know really who he was and I was amazed because I’ve lived in this world for so long. So I thought I’d tell his story, then I’ll move to our story of how we built our intelligent virtual system within our software as a means of intelligence augmentation and the idea of intelligence augmentation is what led me to Doug Englebart, he was one of the forefathers of that. And then I’m going to take you through what we’ve learned in building it and hopefully there’ll be some lessons that you guys can take away. And then we’ll finish up with some questions.
Want more of these insightful talks?
At BoS we run events and publish highly-valued content for anyone building, running, or scaling a SaaS or software business.
Sign up for a weekly dose of latest actionable and useful content.
Unsubscribe any time. We will never sell your email address. It is yours.
So this is Doug Engelbart. Nearly 50 years ago he was actually getting ready to come on stage before a thousand engineers in this auditorium in San Francisco to give a demo and it was a packed auditorium. Doug was absolutely no Steve Jobs, he was a shy unassuming engineer. He actually grew up in a small town outside of Portland Oregon during the Great Depression and during that time he actually went to school as electrical engineer at Oregon State University. He went to Oregon State to study electrical engineering because it was the midst of World War 2 and he knew he was probably to get drafted, and he has kind of figured out that if he was a radio technician radar technician probably less likely to get shot at. Right. So that was his whole thesis of going into electric engineering. He went in there and sure enough he got drafted into the Navy and went to boot camp. Studied how to be a electronics technician he’s getting shipped out in San Francisco he goes under the Bay Bridge on a big boat heading to the Philippines. Everyone in the boat is scared to death they’re gonna have to invade Japan. And that was gonna be a horrific things they’ve heard the news of Okinawa and always hears all these places. And then they hear the seven hills of San Francisco light up with cheers on the boat and the captain walks out and says the war is over. Japan surrendered. So all the guys on the boat scream back to turn it around you know. And he didn’t because it’s the Navy. They went for 10 miles an hour for 38 days to the Philippines. He read a bunch of books while he was there learned a lot came back and finished his electrical engineering degree at Oregon State, went down work for the predecessor of NASA down in Silicon Valley was now Silicon Valley what it was then wasn’t Silicon Valley. He had a job working at Ames Research Center he was driving to work one day he had just got engaged to his wife who was his wife for many many years. And he now had a good job, a steady job, he had a loving wife and ready to live happily ever after – during the Great Depression that was kind of what everybody wanted, if you had that you were golden. But he read this book over and over in the Philippines in the Red Cross library about how to set goals for your career what you wanted to do. So he had an epiphany. He wanted to change the world. So when you started to change the world he said how is he going to do that. And one of the ways the only way he saw to do that was to raise the collective intelligence of all human beings. It’s a pretty bold goal. He was young enough to believe he could do it and young enough to be dumb enough to think he can which I think is also part of the entrepreneurial disease, is you have to have both of those things. And he figured that the best way to do that was use these new things called computers that had just come out and use them as a means of amplifying human intelligence, to increase human collaboration. So. He’s back on stage at this auditorium. He opened his presentation with these remarks and it’s funny to see the presentation (You can watch it on YouTube, we’ll talk about what it’s called in a minute). What if in your office as an electrical engineer you were supplied with a computer display backed by a computer (those things were different in that day), that was alive for you all day was instantly responsive to every action you gave, how much value would you derive from that. He then went on, he stepped on the stage. He had a headset on. They had run 30 miles of wire from downtown San Francisco to his office at Stanford research labs. He had a live video conference. He goes on to demonstrate mouse, a keyboard, a computer display, a graphical user interface with Windows, real time video conferencing, real time document editing, a file system with version control. And all this was 16 years or so 16 years before the Apple Macintosh. Thirty five years before Google or Skype, 40 years for Google Docs. And he really thought that if he did this, he really saw computers as not the tool they were at the time. If you think back in 1968 computers were just massive calculators – in fact they took the name from the people (if you remember the hidden figures movie), they took the name from the people who just calculated stuff all day. They were just massive calculators you’d feed and punch cards they do calculations they spit out these reports, you take the reports around and hand them out to folks who would never read them and then they go back. At the time computers were only owned by the largest corporations and governments. They were the size of a room they were fed by punch cards. He thought if he could show them show the people show the engineers in the audience that these things are more than just calculators that generate reports, these things are tools that can transform the human experience and really improve humanity and help us solve some our largest problems, he thought hundreds of engineers would show up and line up after the talk to help him join his cause. He called the system the on-line system which proves he’s a great engineer, cause that’s a dumb name. And he made the acronym the NLS, which if you really want to go deep on proving that you’re not Steve Jobs, come up with a stupid name and an acronym that doesn’t even match the name. But after that presentation the audience was truly awestruck. They gave him a standing ovation. And Engelbart expected all hundreds to line up and talk to him. After the presentation was over after the standing ovation most of them filed out of the room quietly walked away. Later a reporter who was researching this came back and asked him why do you guys do it? Why didn’t you go? He showed you the future? Right. This was at the 40th anniversary of what’s called the Mother of All Demos. Now they have anniversaries of this each ten years or so. Doug Englebart has passed away now. At each reunion of this, a reporter went through and asked the folks who were in the rooms, he showed you the future why didn’t you do something with it? And it was too big of a leap for them. They had a stack of punch cards to feed into the computers to do what they had been told to do. And they had too much of a day job right. It was too big of a leap for them. Alan Kay, anyone know who Alan Kay is? Invented object orientated programming, one of the followers of Silicon Valley right. He later went on to run Xerox PARC who actually end up hiring away a lot of Doug Englebart’s best engineers actually says this: I don’t know what Silicon Valley would do when they run out of Doug’s ideas. Now if you back up to a little bit earlier, 1962, Doug Newport actually ran experiment where he taped a brick to a pencil. You know this is what DARPA money will do for you when you when you call war scare and all the other things right. But what he was doing was trying to prove a point. The point was is that he studied tools and how humans use tools and using tools is nothing new for humans at all. We don’t have things we don’t have claws so we invent spears and arrows and the spears and arrows not only allow us to do things we couldn’t do but they change who we are as humans as society. This is why when archaeologists go dig up a site what do they look for? They find the tools because the tools speak to who they were and what they valued and what they did. He taped it to the pencil because one of the things he saw was one of the original tools one of the most transformative tools in human history was written language. Written language allowed us to transfer knowledge across time and space. Humans don’t have great long-term memories, we’re deficient in a lot of areas so we create tools to augment our capabilities. This was Doug’s motivation for doing everything he did. And human language – what he found is when you augment human language, written language, when you’ve taped a brick to the pencil and you made it hard to just write sentences, all the higher level tools that are built upon that built to form sentences, coherent thoughts, to tell stories, all those start to break apart. When you make the lower level tools really hard, when you de-augment the lower level tools, you lose all the higher level tools was one of the points he was trying to make there.
This may sound funny. But I sell to banks right. And within a bank within large corporations most of the systems that are built inside those enterprises are not tools they’re tasks. They’re built by the guys who are feeding the punch cards into the calculators right. What they do is, I need to collect data from you to generate a report so I can give to someone else. Right. It’s not a tool that helps you achieve more. At one very large bank (I won’t name the name), for a relationship manager at that bank, to get a deal done there’s 140 different systems that we have to touch just to get a deal done. And when you ask them how many of those are valuable, how may I help you make progress in your life and in your job and trying to build better relationships with your customers, trying to be more valuable to your customers so they’ll in turn be more valuable to the bank. None of them. Their tasks, not tools. So we’ll talk about digital transformation. And this goes back to the innovation theater comment earlier. There’s a lot of banks that we work with, big banks around, who have big companies all around who are under undergoing digital transformation that’s a word that’s also somewhat sullied I think in that is it kind of means everything and nothing at the same time. But I want to talk about digital transformation in the guise of Doug Englebart. Engelbart had what he saw the ABCs of innovation. Have you ever heard of the ABCs of innovation? So Doug broke the world into three activities or processes he called them. ‘A’ processes are the humans and the tools systems that you use to do what you do every day. Right. It’s just the culture or the processes the policies the procedures, how you do what you do. ‘B’ processes are the systems and the human and tool systems that make you better at ‘A’. So that’s the Lean, Six Sigma, the continuous improvement, all the things, the learning management systems, the things that make you better at ‘A’. But Doug also saw a process ‘C’ system. Process ‘C’ system is a system that helps you get better at ‘B’. It’s getting better at getting better and one of the things that Doug saw as unique in humans was their ability to build tools to improve tools to get better at getting better. And this is also why, we’ll talk about in a minute, Doug is known for Engelbart’s law, before Moore’s law, there was Engelbart’s law which was the intrinsic rate of all human improvement is exponential. And the reason he believed that to be so was because they are humans were uniquely capable of getting better at getting better, building tools that build upon tools. Just like language and literature and poetry and all sorts of things build upon the written language that we have, you now can build tools to get better. He called it bootstrapping. Bootstrapping was this process of you build a tool, the tool changes who we are as humans, the humans can then improve the tool system further, that improves the human system, that improve the tool system and it continually gets better at getting better.
Now I like to tell a story of maps in a digital transformation sense. Because if you think about it you can take it all way back to the Vikings. Any Vikings in the room? The Vikings would communicate how to travel through language through storytelling. They would say you need to go to the land of ice and turn left and you hit the Greenland or whatever to get to the US what is now the other half is actually Canada. But then you develop written language and developed mapping tools and they would develop maps and pictorial maps with language on it. And then we’ve done the same and we took the maps and then we came up with G.P.S. so we can actually know where we are on the map right. Then we said also hey don’t we take the map and put it inside the G.P.S.. And that took us to Garmin and now we get we can see where we are on the map. We’ve digitized the map and all that was process B type improvement of just making improving the overall mapping and navigation process. But then you go to Waze, and I think Waze is fundamentally a process ‘C’ innovation as Engelbart saw it. Every person who uses Waze makes Waze better for the next person. It becomes a cumulative effect right. It becomes it’s deep in process C of getting better at getting better with the system inherently has a feedback loop that makes it cumulative which generates exponential growth in value so it makes sense. So this is just to prove Englebart’s Obama’s law. This is GDP for the UK from 1270 on – I don’t know what’s significant about 1270, I’m from the US – but you can see Englebart’s law has proven, believe it or not, as much as Mark likes to complain about the current state of affairs in the UK, there’s probably no better time in the world to be alive than right now. And the thing is as we zoom out on this over any period of time you see the same kind of effect and I think that is Engelbart’s Law in action.
So now I want to shift our journey. So again I talked about how we make software for banks right. I want to talk about our journey to Andi and how we developed Andi which is our virtual assistant. And this is our application back when we first came into BoS. This is how our commercial relations manager might price a new opportunity – 1.5 million dollar deal. As they change anything the screen is constantly popping up these little dots to tell him how to make the deal work better. Right. And this is before Andi – we called this the profitability wizard which was a dumb name that no one but us ever used. Our customers called these the magic dots. And where this came from is, most of the times the system we were displacing was an Excel spreadsheet. And the Excel spreadsheet was highly complex built spent lots of money on. But the RMs if they liked it at all which they typically didn’t, the one thing they did like about it is that it was immediate. They would change one thing and it can immediately see the impact of it so they can easily see what drove the value of deals. Well what happened. What will happen with these sheets is typically the internal I.T. folks at the bank would take over and they said no you can’t have an excel sheet you need a form based application. So they’d spend millions of dollars as they build this application where you fill out a screen hit next fill out another screen hit next fill out a third screen hit next it comes to the last screen that says that doesn’t work. And the RMs gotta go back back back back. What am I going to tell this stupid thing to let me get this deal done? And all of a sudden it becomes a task not a tool. You’ve kind of augmented the entire process.
So we said we can solve that. One of our missions when we started thinking about kind of what is it we’re doing here back in the early days of PrecisionLender was how do we do everything that computers can do well well so that humans can do the things that they uniquely do well, well. So we wanted to enable better conversations between the RMs and their customers right. And one of the things we found is well shucks we can solve for all these things of what it would take to make the deal work. So we put the magic dots on the screen. What turned out to be really valuable is not just showing each of the ways that we show all of them at once. And now every RM immediately knows the relative importance of every deal term to the bank so they can focus on what’s important to the customer and find ways to make positive trades. And this is what unlocks an enormous amount of value. Our our median banking client improved their net interest margin by 29 basis points. Just so you know for a large U.S. bank it’s about a million dollars per basis point per business day in terms of value that creates it on their portfolio of 600 billion dollars. It goes back to business school and one of my accounting professors there who said there’s two ways in the world to make money – you take little numbers and make them into big numbers or you take big numbers and you change it by a really small amount. We pick the latter case of that. So we built this and folks liked it. They never called the profitability wizard. We were even dumb enough to put a trademark by it and I’m making fun of Doug Engelbart with his intellect. Well what happened was we had this guy. This guy is Andy Max. And Andy Max is a wonderful human being who works at the first National Bank of Omaha in Omaha Nebraska. He purchased our system rolled it out and then he would individually work with the RMs and coach them to do better. He just did a deal like this last week. You really ought to think about doing this or Jim did the deal this way you ought to think about doing this and he was being that transference between and kind of cross pollination and Andy would call us up and say I was just working with this RM and he had this situation – could you add a magic dot to the screen that when you see him doing that, nudge him this way, tell him they ought to think about this. And we were all sitting in my office I’ll stock I think it’s one of our thing was Marcy was talking to. One of our engineers. And we were working through that list latest list of magic dots from Andy. And I told Marcy I said Andy makes our product a hundred times more valuable to that bank. Wouldn’t it be great if every bank had an Andy and that was what led us to… well shit, why don’t we do that? Why don’t we try to give every bank an Andy, could we do that? And so this happened two years ago almost when we had the idea and then we started building it and in January 2017 we launched Andi and we said every PrecisionLender user now has their own pricing analyst. Her name’s Andi she’s doing the job she learns quickly, she works 24/7, never sleeps, she sees every deal at the bank the entire portfolio how it’s evolving and she monitors your relationships and alert you to threats and opportunities of things you can do and you can also ask Andi questions and she’ll give you answers and guide you through how to use the software and all this stuff. And the other thing that we made it is completely extensible, we completely copied Amazon in this respect, in that we had an Andy skillset right where you can actually build your own skills for Andy. So all the things that Magic dots that Andy Max would ask us to add, he can now add himself you can add a quick little skill as if you see him doing this till you do this. Now it turns out we thought we first did this we thought we’ll be able do some really fancy A.I. stuff because we have a lot of data right now. We price about 600 billion dollars worth of commercial deals through PrecisionLender each year and that’s doubling about every three or six months. We see an entire portfolio of commercial loans, deposits, and other fee based business at banks. That’s about 250 billion which if we were a bank we’d be the fourth largest bank in the U.S. slightly bigger than Citigroup which is kind of amazing. And when we first thought we said we can use the data we have to train things to predict churn to predict the utilization of lines of credit to predict which sort of products for a customer like this in an industry like this with a relationship like this at the bank, what sort of things might they might they find attractive that they were in. We talked to them and what turned out to be more valuable I think was some of the really mundane things the things the AI nerds, the data science folks might turn their nose up and go that’s not really AI, that’s simple heuristics right. It was if you see him doing this just tell him do this tell him to consider this. And those simple tactical kind of coaching them through using the application turned out to be enormously valuable in the application.
So what have we learned? And this is the part where we’re trying to give you the takeaways of the case study and things we’ve learned. One thing I’ll tell you, two things. If you want to know how to start a business selling into the enterprise I would say start at the customer, find a business that makes good money right because if you’re selling to somebody who doesn’t have any money that’s gonna be a difficult slog. But find a business that makes good money, start started their customers work backward into that business. When you first hit the biggest most gargantuan spreadsheet stop there, build a SaaS application. Right you’ll you’ll do really well. The other piece is when you build that SaaS application find your Andy. Ours was Andy Max and then take that person and embed them in the software. And we actually saw Andy two weeks ago and talked to him and thanked him again for guiding us down the right path of showing us the example. But actually trying to find a way to translate it, how do we put Andy in our software right. Is really what a lot of what we do.
So what have we learned. So one context is king. You have to narrow the context, and user interface and user experience matter immensely. Now any kind of A.I. machine learning whatever it is basically an idiot savant. It’s very good at a very narrow thing but it’s not good across things. There’s actually a theory in AI called the no free lunch theory which exactly says this – it can be good as the narrow thing but not good at a lot of things right. And as soon as you try to make it wide it gets very difficult. If you narrow the context… Andi is not Alexa or Siri, Andi can’t book a movie for you or anything like that but Andi can price the snot out of a commercial loan right. And once you narrow the context things get really easier. So if you can narrow the context it helps a lot. The second thing is the user interface and particularly user experience matters a lot. You notice we had to have that real time feedback so as the RM is going through and making changes in the application and we thought about it this truly as we personified Andi into the app we said we need to be able to see we need to be them look over their shoulder. I don’t mean to see in the pixels. So every time you change if you take the loan amount if you change the rate if you change the collateral if you add in deposits or bring in other fee based business, Andi sees every move they make and can correlate that with the outcomes they produce right. So now you have you can see the full user experience you can see the full everything they make do and ground that to outcomes. That’s what gives you labelled contextual data that you can use to actually coach better solutions. Not all Data’s created equal. This is the second premise, that labeled behavioral data is most valuable. If you want to train a system to make suggestions to the users you have to you’ll see what the users are doing to see what outcomes are produced. That’s the labeled bit of the data. And the behavior – what behaviors led to that outcome. So that I coach them on different behaviors. Most of the data that exists within banking within large corporates is not this and no amount of big data projects are ever going to fix that. So if you think about like with Waze right Waze every interaction makes it better, every user every interaction makes it better for every other user. In our system every opportunity that’s priced every relationship that we’ve seen makes it better for every other user. How do you get that process ‘C’ loop going and you have to go to ground it to label behavioral data. And I think ultimately you have to build it organically and the only way you can build organically is to narrow the context. Right. As a practical matter.
Nobody actually wants artificial intelligence. They just want intelligence. And I think this is incredibly true. None of our RMs give a darn that it’s whether it’s A.I. or not. Right. The guy in the smug dude PhD with a coffee cup standing here doing all that’s not really AI. They don’t care about him. Right. And it is typically him so no feeling bad about that. You want a simple heuristic driven insights are really powerful they drive early value they establish delivery pathways which are most important I’ll talk about that in a minute and they establish success metrics and set the bar. So they establish like if somebody later wants to do a recurrent neural network deep learning system where you got gotta do better than the simple heuristic, if you can’t do better than simply heuristic, stop, right. So at least you’ve set the bar and then you can go fine. So we actually could find who are the folks who manage relationship matters within the bank, who were the best coaches. What do they tell them to do. Right. And then we just see what works for them. See what works best and spread it to the rest is this simple equation and folks find that quite magical and that’s what we call amplify the human in the loop. And this is where I come back to the title talk build Ironman suits not Terminators. How do we augment humans to make them better? Not try to replace them in displace them because there are actually there are a lot of things that humans do uniquely well that machines don’t do well. And by the way if you want to know what things those are, really if you look at our education system it takes us 18 years to learn mathematics. Right. Computers learn that quick. But when we’re three years old we can catch a ball and we can converse. We can understand emotional sentiment. What’s really easy for humans is really hard for computers what’s really hard for humans is really easy for computers. This is chocolate and peanut butter we need to make put this together and make it work. So build Iron man suits is not Terminators.
The last thing is I’m an engineer I have electrical engineer which is the joke is you can’t have a geek without a ee. The converse is also true but more difficult to prove. But we build Andi and Andi truly is marketing it’s photons neurons just a way of framing it and thinking about it and it’s amazed me. It is relatable and human. When I go in and talk to a roomful of RMs and I talk to them about machine learning and all different you know conversational interfaces and blah blah blah. I tell him Look Andi. Andi is going to help you and Andi is going to see everything that’s going on and help cut you to a better outcome and they’ll get up out of the room and go Oh God I need an Andi. You know if I talked about machine learning they would just glaze over and go to sleep and I’d lose them. Right. The other piece. We’re big fans of jobs to be done. How many of you do jobs to be done. It really is a fantastic way of you know so… No one wants a drill bit. They want a hole. So why do you want a hole, to put up a light. Well, why do you want a light? I wanna put a lamp by my bed. Why do you want a light by your bed? So I can read in bed. How about I give you a Kindle with a backlight. And once you go through the five whys or so you can start uncovering the job to be done. What is fascinating to me about the personification of Andi is we used to have a proliferation of questions, of feature requests, and Corey here can tell you about these – folks, could you put a button here? Could about put a button here and a report that tells me this? I need to report on our dashboard that tells me this. And then what you do is you’d ask him Well what do you want a button there? And why do you need that report? Why do you need that? What are you gonna do differently once you know what the report tells you? Why don’t we just have it do that? You know. The mental hack that happened when it’s personified is they don’t tell you to put in that put a button there, they ask could Andi do this for me? Can Andi do this for me? And it actually cut straight to the job to be done. It’s almost like this human hack that takes place in their brain. And it cuts straight to that. And the last thing is it humanizes the machine instead of mechanizing the human. And I think that is something that you really ought to work to do. I asked this question of folks and I’ll ask it here how many of you concerned that AI is going to destroy jobs and displaced workers? How many of you are concerned that I will displace you from your job? Mark..0. Well. Typically when I ask this there’s a lot of hands go up for the first one and not many that go up for the second one. And what you find is that well it wouldn’t happen for me because I’m a knowledge worker. I’m truly doing human work. Well who are you concerned about? You’re concerned about the folks who are doing repetitive manual labor that robotics will take out of there. You know that you worry about them losing a job but in reality were you worried about them having a job that really wasn’t fit for a human to start with. Right. I thought about this with somebody recently I said we used to live in a world where we would interview humans to see if they’re worthy of a job. I think where we’re going to head to is a world where we’re going to ask ourself continually Is this a job worthy of a human. And I think that’s an interesting parallel like the fact that somebody is doing the same thing all day every day. Is that a job that truly is worthy of the dignity of a human? It’s just a different way of thinking about it that’s less fearful and probably won’t make it into the news because of that.
Here’s the cool part I want to talk about there is an intelligence augmentation value chain or an AI value chain. And to win you have to implement the entire chain and most projects die at the last mile of A.I.. But what that means in a minute. This is a guy Ajay Agrawal. He’s a professor at the University of Toronto, Economics professor who studies A.I. systems and those things and he maps out what he calls the anatomy of a task where you take data and you generate a prediction. That prediction combined with human judgment leads to an action, that action produces an outcome. But you then look at the actions and the outcomes and feed it back to make better predictions. And so he has a great paper on this describing the anatomy of task and what when computers first came out they make calculations almost free now with machine learning, prediction is becoming almost free. And that actually moves the value down the chain that whenever you have a value chain whenever the value of a complement falls dramatically, the value of its other complements go way up. Right and we’ve seen this throughout history. Next one is all time when I got him Gordon Ritter Gordon Ritter’s a partner and a founder of emergent Capital Partners out in Silicon Valley and a good friend. 20 years ago his firm is small and thesis driven. He’s not an investor in our business but a good friend his firm is thesis driven. His first thesis 20 years ago was SaaS is going to take over the enterprise. That was his thesis. He invested a million dollars the first million dollars into a little company called salesforce.com and did rather well. His second thesis about 10 years ago was that the first wave of SaaS was horizontal. Salesforce, NetSuite these things the next wave of SaaS will be vertical and it’ll be far bigger than the last. He put six million dollars on a 20 million tree into a company called Viva Solutions. Viva went public seven years later at 6 billion dollar valuation. I know Peter Gastro the CEO there through Gordon quite well and that was a vertical SaaS solution built for the life sciences industry. So like GlaxoSmithKline those guys use it, highly regulated, they built a vertical specific solution. His current thesis is what he calls coaching networks or the coaching cloud and what he says is that you’re going to see these networks emerge where people just do what they do. Right. The machine sees what they do does what it needs to do with that data. And then coaches them to do it better. And so you’ll see this gathering, comparing, coaching and then you see what the human does and you feed it back into the loop so you gather what all the humans do, compare it to what the best humans do grounded outcomes, coach the other ones to be better switch back to aggregate all see which actions are taking place and feed it back. So one of the examples of Gordon’s companies is a company called chorus.ai, has ever heard of this? So what Chorus does, is it listens to sales calls, understands the language, extracts out to do’s and next items and follow ups, fills in Salesforce the CRM system for the salesperson but then coaches the salesperson or what they could have done better based on what is seen other salespeople do. So as a human you do what you do best, the machine sees what you do compares it to what others do and then you to be better and remove friction from the entire process and amplifies the human. I think that’s a great example of building Ironman suits not Terminators. By the way I talked to Gordon about this and we talk a lot about coaching networks because I’m a big believer in the thesis. I ended up explaining this to somebody about two weeks ago, his first thesis 20 years of the enterprise that was going to take over I think was right. I think we all agree with that. The first wave was horizontal. The Second Wave which built upon the first was vertical. Because you can really add value to domain specific. The third wave and I think the final wave will be personal. Right. And it’s built upon the vertical piece as well, personal meaning I’m going to coach you how to be better at what you do and I’ll coach you differently. But within that vertical domain so they build upon each other and it’s interesting to think about it goes from horizontal the vertical to personal and I think that that’s a helpful way of thinking about it.
This is this is a guy named Jerry Chin who’s at Greylock. He talks about systems of record, systems of intelligence, and systems of experience. Systems of record are the ones you’ve seen around quite a bit. They’re the three letter acronym folks that captures data about what happened. It deals in facts. This is a serial that your CRM the ERP the SVP all these things and it captured what happened and when and just the facts right. Systems of intelligence transform facts into beliefs. Now beliefs are not binary they’re not either true or false. I’m 95 percent certain this customer is going to churn, I’m 50 percent certain this customer is not going to pay back this loan. Right. Whatever the certainty is that they have, they’re fuzzy right. Predictions are just beliefs about the future. That’s the only difference. If is a belief about the future it’s a prediction. The systems of engagement are the ones that ride shotgun with the end user while they do what they do and they transform the facts and beliefs into measurable actions right. That based on what I believe based on the facts and the beliefs I think you should do this right now. I think you should talk to them about how you’re doing a loan to a shipping industry to a customer in the shipping industry. We have a wonderful foreign exchange service business at the bank. You should definitely bring in some of the FEx team to talk with him. Right. That is transforming facts and beliefs and actions and then you measure that feedback like Agarwal talked about. Now I think where the early days of this and when you’re in the early days of something there’s many ways of saying exactly the same thing because everybody sees the same thing of the shadow of the same thing and no one knows what to call it. And if you look at this. You can see Greylock’s Chin calls it systems of record, systems intelligent, systems of engagement. Satya Nadella at Microsoft calls it perception, cognition, and action. Gordon Ritter calls it gathering, comparing, and coaching and then Agarwal calls it data, prediction, and action. They’re all seeing the same thing but calling it different things. And that last mile, the translation of insights into action is really where most things die right. I’ve seen lots of banks, big banks hire a bunch of data scientists and they do elaborate models that make predictions and then it goes nowhere. There’s no pathway to deliver that. What we talked about a lot of times with Andi is how do you put the smallest bit of information on exactly the right eyeballs at exactly the right time. Where it’s going to have the most impact to the business right. In that last mile of A.I. which is that delivery mechanism is often missing. So Centaur Chess. In 1997 IBM’s Deep Blue beat Garry Kasparov in 20 moves. He immediately demanded a rematch. IBM said no because their marketing was done. And then they kind of shut down the whole thing. What’s worth noting is the current laptop chess programs are probably more powerful right now than deep blue. Your iPhone 10 is 30 times more powerful than deep blue. Which is worth just from compute power standpoint… It’s pretty amazing. After this Garry Kasparov created this thing called Centaur chess or freestyle chess and what that is is now instead of humans competing with machines or humans competing with humans. Now you can have humans you can have machines or you can have human and machines working together and competing. And what turned out was that was a bit surprising. It wasn’t surprising at all that the best machine beats the best human because after Kasparov has lost the best machine always beat the best human at chess. But what was really surprising is the human plus machine beat both the best machine and it beat the best human. So the human and machine pair actually ended up winning. And what’s also mentioning, it wasn’t the best human with the best machine. It was a moderately good human. With a moderately good machine she actually had three laptops, three machines and a really good process for how to work together. Right. And this is where when you’re building a human plus AI team the hard part isn’t the AI, it’s not even the human, it is the plus. How do you put these things together to make them more effective and how do you find a way of blending these two together? It was a mid-level because chess players are ranked Grandmaster all although there was a mid-level chess player amateur chess player with three laptops. And when the laptops would agree he would just do the what they told him when they disagreed. He would actually run some scenarios on the laptops and ask them more questions. So one of the things out of this is that computers are useless. They only give you answers which was probably it was mostly right on that… Computers are great at giving you answers. But humans are good at asking the right questions. Right. So allowing those two to work together is really quite powerful and ultimately freestyle chess ended up proving that.
Want more of these insightful talks?
At BoS we run events and publish highly-valued content for anyone building, running, or scaling a SaaS or software business.
Sign up for a weekly dose of latest actionable and useful content.
Unsubscribe any time. We will never sell your email address. It is yours.
Now again there’s many ways of saying the same thing. When you look at that full value Chain right there I showed you Gordon Ritter’s and a Ajay Agarwal’s.. Gartner calls this cognitive expert advisors which is a very Gartner kind of thing to say. Accenture calls it citizen AI, Gordon Ritter calls it coaching networks, Deloitte calls it cognitive collaboration. It’s what they call that complete cycle again. I think multiple people are seeing the same thing just calling it different things. But I like calling it applied insights or coaching and we think about Andi as a platform for Applied insights we think about PrecisionLender as applied banking insights or you have to have the vertical piece to be able to apply insights about what a coach you how to play basketball have to know how to play basketball right. I can’t just build generic coaching platform it has to have the vertical piece but the key parts about coaching is it has to be contextual. Meaning I have to understand the context. If it’s basketball if its banking is what I have to understand that context. It has to be contemporaneous. I can’t tell you a week from now you should have done something better with you know it has to be in the moment. Hey. If I’m coaching on golf you’re setting up wrong on the ball you might want to change your stance right now to hit it better right. That immediate feedback is something that that humans react quite well to. It has to be constructive. I have this debate I have to explain this to some higher up folks at the bank. Sometimes you notice that Andi gives you suggestions when you click and they pop up right. They’re not always jumping in your face you know. And by the way you’ll notice when it says Here’s all we make the deal work. They’re all green. I could have changed two lines of code and instead of here’s how the way to make the deal work and made them green they could’ve been here’s where you screwed this up and made them red. Functional requirements 100 percent met either way, the human impact is dramatically different right. So understanding it has to be constructive because I’m trying to coach humans to a better place. Individualized – How I coach the peewee league on how to play basketball the 11 year old team versus how I coach Michael Jordan or how my basketball is different. So you have to customize it to each individual based on what we think so the most helpful to them. Actionable – and then the last one is actually we try to make it so. Hey if I know what to do you click it and we do it right. I’m not walking through something if you say Yeah like do that you click it we make it happen. And the last one is attributable – Is that when we build skills inside of Andi those skills you can actually see how often they’re shown. The impressions. We know how often they’re clicked the click through rate and because we’re grounded to the outcome because we have a really the perfect use case for this, we know the net income and return all capital of every action that’s taken. We can ground that to outcomes so we can actually attribute if you build 50 skills, here’s the ones that are really being engaged with by the RMs and here’s one that are driving value at the bank. And that attribution is really really helpful in terms of closing that learning loop. And again I think it all comes back to Doug Engelbart right. How do you get to process ‘C’. How do you get better at getting better. How do you build a system that inherently forces you or gives you a pathway to get better at getting better.
And so I end with this which is the same thing he started with 50 years ago. Which is when I first read this I’m like Holy mackerel this is what I just figured out two years ago that we should be doing. Something that I think is something we find like the punch card guys who use computers as calculators to generate reports are assigning tasks to folks. What we need to do is build tools. Tools that allow us to not only improve what they’re doing but to raise what humans are capable of. So that’s all I have. Thank you guys.
Audience Member: Thanks for the talk. You talk about how difficult is to get the action part and in your case if you had enough time and money would you be able to get an Andi that didn’t need a human operator or do you see a human barrier that can’t be transposed no matter your effort.
Carl Ryden: So the question was could I see you get to where there’d be no human in the loop. I think that’s going to be a lot for our particular use case I think it’ll be quite a long time. Because there is a human aspect of commercial lending in particular where you’re dealing with a business owner. So one of the truisms about commercial lending is you’re fundamentally selling a product no one wants. No one wants a loan. Wants indebtedness. No one wants their financials to be examined and underwritten and judged right. All that’s painful. You ask folks you know what do you want. They say I’d like it faster and cheaper. Well of course that just means they never wanted it at all. What they want is what the loan enables them to do and what having a human being able to connect to that job to be done. That the reason you want to buy this piece of equipment they really need a loan for as piece of equipment is so you can actually expand your business and get home to your family and see your kid’s soccer game. That’s the job they’re hiring the loan to do. That’s the job they’re hiring the bank to give them. And so I think there’ll be a long time where humans are in the loop on this. Just like by the way computers I think are quite good at medical diagnosis. But however I would not want to be told my medical diagnosis by a machine. You know there’s a human conversation that requires the things that a four year old or a 10 year old or good at that it’ll take forever to train the machine to be good at it. And I think ultimately if you give me a good relationship manager who can fit the Iron Man suit, they will always beat the Terminator. I may be proven wrong many years down the road but I watch a lot of Marvel movies with my kid, with Pete here, and it seems always work out that Iron Man wins.
Audience Member: And if I may ask a rather dull question relating back earlier to the discussion about patents. Is there a kind of land grab here where everyone’s just going this with AI? Or is it even possible for people to patent a ML models or is that something that’s not so…
Carl Ryden: I don’t think so. I don’t see many a lot of it. A lot of the. The second phase of that assistance records is intelligence. A lot of that stuff even the big guys have learned that the rate of improvement happens faster in the open source world. And by the way if you go read the ABCs from Engelbart one of the things he talked about was process A you’ve got to have those and have good process of how you do what you do. Process B can be internal to your company. But often the best source for process C getting better getting better is community. And I think even he predicted the rise of open source before because how do you increase the rate of getting better at getting better. If you open source those models. Even the biggest companies Google and Microsoft and Facebook and all these folks are open sourcing their models because you get better at getting better faster. And so I think that’s the trend that’s going to happen now. My personal belief… in the US it’s actually black letter law that you can’t patent a mathematical algorithm. Somehow people still do. And there’s getting a patent and defending a patent are two different things. And ultimately what I think how you build the competitive advantage is you get on a trajectory. You get the flywheel spinning where. So wait Waze doesn’t need a patent. Right. If I have more data flowing in that allows me to make better decisions. This is a concept I said I spoke at the Microsoft Azure client advisory board in 2010 when it was first starting and I said statistical significance is the economies of scale of the information age. If you get to a better answer you have a you have an advantage which is very difficult to replicate. So once you build a system that can actually provide better results and better outputs, that’s the defensible position and building that ecosystem of that data flowing through there I think is the most defensible. I don’t trust patents. I’ve been an expert witness of all these things for taking them down. You spend a lot of money a lawyers that they’ll gladly take it in patent anything you want whether or not you can defend it. You get spend more money on lawyers later. So I hate lawyers except my partner who is a recovering lawyer so.
Mark Littlewood: Can you tell us a little bit about the background of PrecisionLender. Rather than AI I think one of the really interesting things about your organizations is that it slightly bucks the trend because to be a fintech business you need to have tons of cash and you have to have a really really strong balance sheet which is why people have to take venture investment to even get it going… Discuss?
Carl Ryden: So there’s always a reason right. I mean that back in the day to build a SaaS solution you had to build your own data center so you needed tons of cash. Then when Amazon and Azure and other things came out well you don’t need tons of money to build a data center you’d be a fool to do so. Right. So what you need cash for now. Right. And so you need money on the balance sheet you need safety, security. But also for a lot of SaaS companies that’s why B2C SaaS companies or require their customer acquisition costs are really high and they earlier they talked about marketing and throwing marketing dollars at it. At some point if you’re in the venture business you have to have companies with strong growth trajectories with high capital needs. Or your business model disintegrates right. So you have to have high capital needs according to them… this is a key part. I was in the venture business my business partner Ken was in the venture business. We were both successful we sold companies before. We started with the idea that we were never going to take venture money. The caricature was they’re all assholes you want nothing to do with any of them. Because I knew them and I’d seen them and they’re not all but you know I knew enough of them. In a few weeks I asked individual business what do you do. I said Well I make small companies perform unnatural acts. Right. It feels like you know. And that’s the name of the job. Right. And so we decided we wouldn’t do that. So we got to about I want to say four million four and a half million recurring revenue and we’re about 15 employees and as a founder I’ll tell you. We generate cash throughout the life. You know. Ken and I both worked for a while without any salary to get to that point but we were generating cash. We’re paying ourself at that point but we constantly felt that we were sizing the business. We’re sizing the opportunity to the business not sizing the business to the opportunity. And the thing that changed for me was when I went to the Christmas party and we had 17 people and their families come to the Christmas party and you think this will be like a wonderful thing and Peldi you probably know this feeling. I’m sure you do but you you see all the folks at the Christmas party with their families and at first that was great. What a wonderful bunch of folks. Then you go Holy shit. If I screw up these are the folks who I’m going to inflict harm all right. And then you get this dread and like Christmas parties became like a horrible day. And that’s what I know. Okay look I got to solve this problem that we’re not. So we actually have we would say no to all the VCs. And then one kind of finally broke through our barrier and end up talking to Ken. And we’ve started they’re all assholes. Oh but these ones aren’t. They seem to be pretty good folks so we did half primary half secondary… And the key to raising venture money is to never ever need it. And then you get to control kind of who you pick and you pick your partners and you pick your partners to solve specific problems and you write down the job your hiring them to do. I want to de-risk it to myself, to my family, to my employees, and to my customers. Right. And so we did half primary half secondary secondary for those I don’t know means they bought shares from us and gave us cash, the founders. And they put the primary means they put cash on the balance sheet. Now about a year later. After we launched Andi. We got a mentor of ours introduced us to getting Justin Lafayette who runs Jordan Partners up in Toronto. And he says Justin is the best board member I’ve ever had and they have a strong AI focus collected universe to Toronto. He’s an entrepreneur. He says you need to meet Justin. I met Justin. I love Justin and his firm, they love what we were doing, we connected and then we did a half primary half secondary at a different point. Again same goals. And then just last fall we reached another point with over 100 employees we’re expanding internationally. And we had another friend at Insight Venture Partners Richard Wells who we had known from way back. They love what we were doing want to be part of it so we did all secondary round with them. And the goals for that and I wrote this down and I sent it to all the board members in our senior management and to Richard. I said. Our objective of this is number one. Do no harm. We’ve got a great company a great culture we’ve got a great board. I’m not going to want to screw that up. Check. I know Richard I’ve known him for a long time. Number two is I want to get everybody aligned that we believe we can make this into what it needs to be from the standpoint of growing it for the benefit of our current employees as well and I want to get everyone aligned around that because one of the hard things if you have multiple venture investors and they’re misaligned. Somebody wants an exit in one year’s money was it three years and all that. I’ve seen that so we said everyone get aligned around this. So we did that and then the third piece was find us a partner who can help us and see around corners who’s been there done that who can bring stuff to bear to help us as we expand internationally and otherwise. And they checked all the boxes and I sent it not just my board I sent it to Richard. I said if you don’t fit this bill this’s probably not gonna work out for us and this is what I believe. Tell me if I’m wrong and it turned out to be really really good. So even though we started with all of them are a holes they’re not. Being able to pick and choose the ones you want but then be very explicit around what’s the job I’m hiring them to do, and what the expectations are. And by the way, at every presentation I ever did we had two tells. So every presentation every day it started with I would show them the values. Right. And if any of them were like fast forward through the values I want to see the numbers I said like well we’re done. The second thing was when they’d come in for a meeting with us we typically have lunch or muffins or coffee or whatever. The ones who would get up and walk away and leave and catch their Uber to the airport and leave a mess for us to clean up, that was our tell that that this is about them. You know they’re not helpful which is one of our values. So it’s one of the things. We met with Justin and we love Justin. Ken and I both thought please pick up that cup please. That was I was one of our little tells, and he did. He picked it and took it in there put in the dishwasher and I think it’s a good tell. The other tell I’ll tell you if you ever if you’re a technology company and you have a venture firm and then they hire a third party to analyze your technology, that’s a negative tell I would tell you. If they’re not confident enough that they can make that call on their own. Or if they’re not confident that they make the call their own you’re in for a world of hurt. So. Just. Another safety tip.
Audience Member: You opened with the ??? piece. And you’ve just referred to it now. And what I’m finding interesting at the moment is the number of places with companies using ML where they’re having to make internal values decisions about whether or not something is the right thing to do and legislation is not going to help us here. So does that arise for you? And how do you handle it with your technical team?
Carl Ryden: We have our values. We have operating principles. And then we’re working hard to build kind of the Andi design guide for what we’ve opened up for the banks to build their own. So we made the choice to make them green and make them positive constructive. But we have an educational thing incumbent upon us to design guides of how Andi doesn’t seem in one minute helpful and friendly another minute like you’re gonna be fired. And so it’s incumbent on us to put that forth. The other piece is why I think having a human in the loop mitigates a lot of that. So ultimately Andi makes suggestions but the human judgment has to come into play on what actions are taken. And that gives us a huge mitigate so we can actually see if we’re offering actions that are not being taken why. If we’re doing something different than what we’re offering, why is that? But I think for us putting a human in the loop was our main guard rail against that sort of thing. But there’s a great company called Integrate.ai that’s also a fellow Georgian partners portfolio company, a lady named Catherine Hume who you ought to have come speak, she’s amazing. They do a lot of work on ethical AI because they’re doing more automated stuff without humans in the loop at all times and they’ve done a lot of work around ethical and explainable A.I. and in how that comes to be.
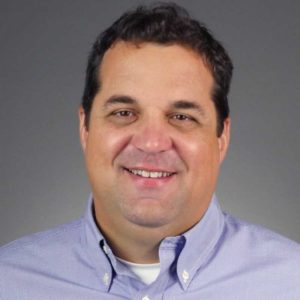
Carl Ryden
Beginning in 2009 with a blinking cursor and a blank screen, Carl developed PrecisionLender’s loan pricing system. He still codes but also provides strategic consulting to PrecisionLender clients and thinks a lot about artificial intelligence in banking.
His breadth of experience and passion for technology, finance, strategy, and software development give him a unique perspective on risk-based pricing. He has an MBA from MIT Sloan, Master’s in Computer Engineering from MIT and a BS in EE from NC State University.
All his degrees didn’t teach him the single most important thing in the software business – it’s all about the people.
Next Events
23-25 September 2024 🇺🇸
Raleigh NC
BoS USA returns to Raleigh NC
31 March – 1 April 2025 🇬🇧
Cambridge UK
BoS Europe returns to Cambridge UK
June 2024 🧑🏼💻
The Road to Exit: A BoS Mastermind Group
Facilitated by Mr Joe Leech
Want more of these insightful talks?
At BoS we run events and publish highly-valued content for anyone building, running, or scaling a SaaS or software business.
Sign up for a weekly dose of latest actionable and useful content.
Unsubscribe any time. We will never sell your email address. It is yours.